According to Gartner, 50% of businesses looking to roll out AI-powered systems in 2023 will get stuck at the proof of concept stage.
So how can you be part of the half that will successfully launch your AI project this year. The key is to understand that delivering a successful AI solution requires a whole business approach. In addition to understanding and filling the appropriate technical roles, you will need to set up the internal reporting structure that will enable a smooth development phase and successful roll-out.
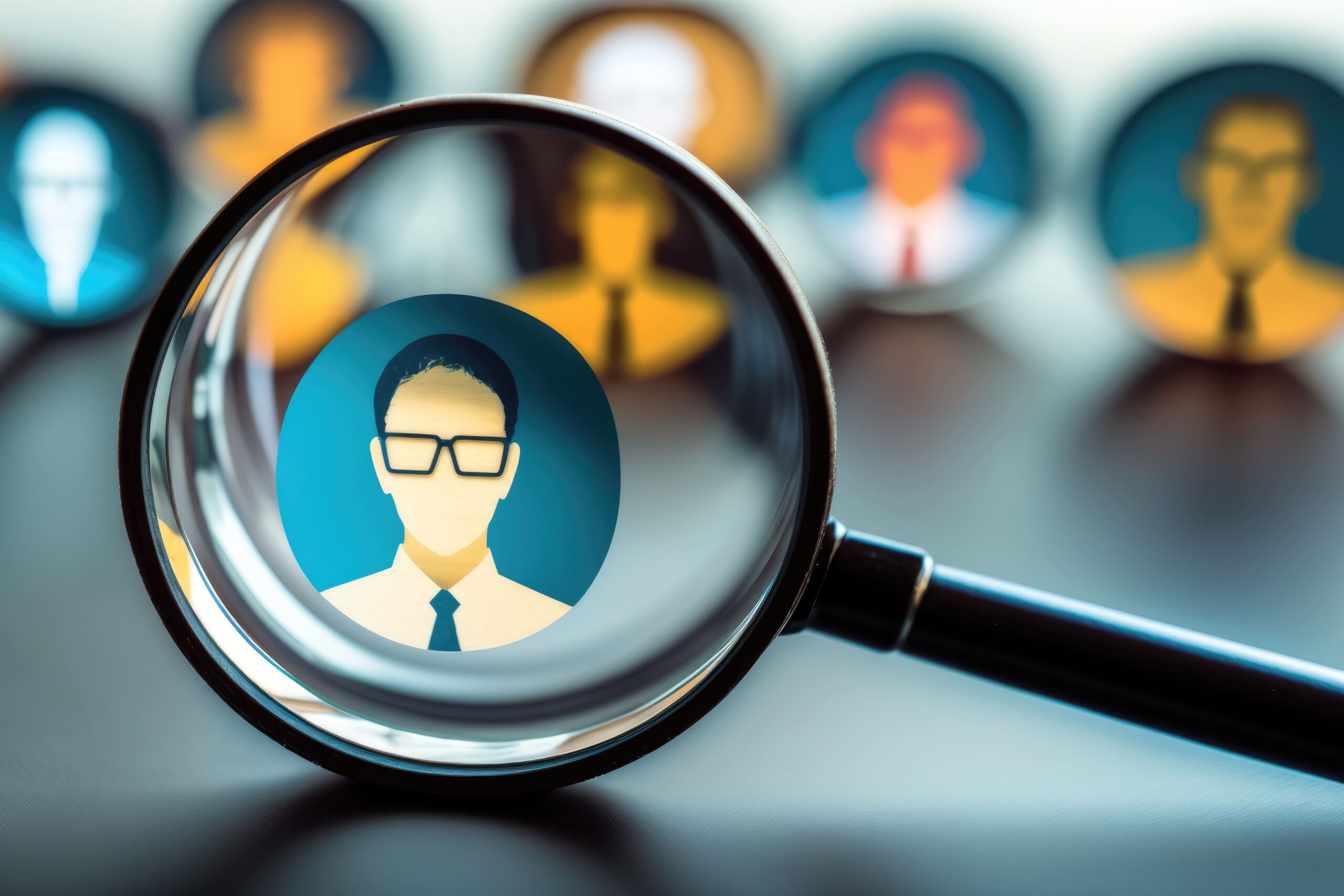
Three domains of expertise
There are many ways to slice the AI project cake, but it can be useful to break down an AI project team into three domains.
At the top level are the roles responsible for translating the needs of the business into a relevant model architecture running on the best quality data. The two key roles in this domain are:
- Data scientists. The data scientist's main focus is on analyzing and interpreting data using statistical and machine learning techniques.
- AI architects. The AI architect's main focus is on designing the overall architecture and selecting the appropriate AI technologies to meet the business requirements.
At the middle level are the roles responsible for actually obtaining and cleaning the data and designing the AI model itself.
- Data engineers. The data engineer's main focus is on the data infrastructure and ensuring that the data is properly prepared, cleaned, and stored.
- ML engineers. The ML engineer's main focus is on designing and deploying the ML models that will analyze the data.
At the bottom level, there are the operational roles responsible for deploying the model and monitoring its performance (MLOps)
Joining the dots
Recruiting successfully into the positions outlined above is clearly important, but it is just as vital to ensure that each domain is connected together, and that the AI project as a whole is plugged into your business structure.
This includes both at the top level, with strong links between the C-suite, data scientists and AI architects, and the bottom level, with MLOps working together with DataOps, DevOps and other IT specialists with in-depth knowledge of your business IT systems and processes.
Finally, there needs to be cross-domain links with non-technical teams that can inform the project leads on matters of data fidelity and operational feasibility. For example, building an AI-driven accounting system will need input from senior managers in the finance department. They will have the most relevant knowledge about which data is relevant and how the AI deployment will affect their performance.
Time to recruit?
Once you are confident you have set up a reporting structure that works for you, it's time to fill those technical roles with the best candidates in the marketplace.
Breaking down the roles into the three domains described above can help you to design suitable application pathways. For example, it should be clear that data scientists and AI architects will be required to collaborate closely with the CTO and therefore should have appropriate strategic thinking and communication skills. An AI architect with sound business sense will more readily understand how to translate the business's need for profit into a cost-effective AI design.
For lower level roles, the application process can focus more closely on strong technical skills because the Operations roles will be required to work quickly and accurately to ensure the AI models run smoothly and bugs are quickly reported and solved.
ML engineers (who are expected to be in huge demand as AI tech progresses) will need a wide range of technical and soft skills in order to successfully translate the AI architect's designs into reality and ensure a continuous feedback loop.
Are you looking for a recruitment partner in the IT industry? Please contact Myticas Consulting.